Most Model Predictive Control (MPC) systems have a fixed plant model (i.e. does not change) and hence represent a plant state at a certain operating point or an average operating point. We know that operating plants behave differently as the plant load changes. For example, the relationship between a temperature and product quality will be different when the plant is at 50% load than when it is at 100% load. This nonlinear behavior is intrinsic in all operating plants. So how can a linear MPC work with a plant that is intrinsically nonlinear?
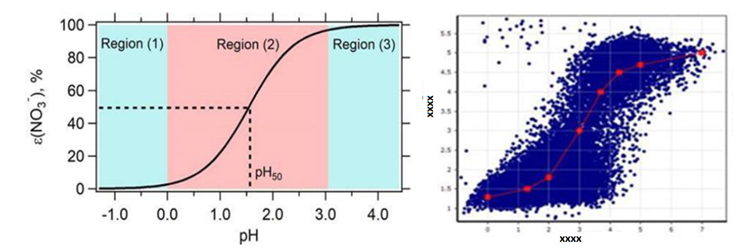
Can linear models represent a nonlinear plant?
Process models for MPC are developed by making perturbations in a process at an operating point that represents the norm for that process. Data collected after making perturbations is analyzed and developed into a model describing the relationship between different process variables. By nature, the model is fixed and therefore can only represent one operating point, irrespective of the actual process behavior. Hence, there is a mismatch between the MPC model and the actual process. The model being a linear representation of a naturally nonlinear process. A linear model cannot represent a nonlinear process, so how can linear MPC work in a world of nonlinear processes?
How does linear MPC work?
The perfect solution for representing a nonlinear plant would be to create a nonlinear model or a “pseudo” nonlinear model by creating several models to represent several operating points. Although these options are possible, they are not practical and are costly. The widely accepted approach to implementing MPC is to create a single, linear model of the plant at the “normal” operating point. For example, a model could be developed at 70% load and another at 100% load. Typical refining and petrochemical applications can operate with a single or a maximum of 2 plant models. Certain processes, such as polymers, have well-defined operating points and are best suited for a nonlinear APC application. Factors that determine whether a nonlinear model should be used or multiple linear models are: ease of deployment of the solution, cost of technology, ease of maintenance and training. Remember to always think about the business case: Assume that a plant runs only two weeks per year at low load conditions that a single APC model cannot cover. Why not switch off the APC application and let the operators run the plant for these two weeks? It might help to keep the APC application simple (and cheap).
Keep in mind that a (small) model error is not the end of the world. The success of a linear model representing a nonlinear plant is because the error between the actual plant and the MPC model is provided as feedback to the MPC system, so the model is “corrected” at every execution interval of the MPC system. This error between the actual plant response and the fixed MPC model is called prediction error. Since most MPC systems execute at a frequency of around 1 minute, the feedback mechanism takes care of the prediction error. Hence, plant nonlinearities can be dealt with elegantly. There are also filtering mechanisms on the prediction error, which can further improve the performance of the MPC system. If the nonlinearities are severe, then a second model could be developed, or a nonlinear model could be considered.
Fast and easy deployment of MPC, quick benefit realization
The methodology of having a linear MPC model represents a nonlinear plant is tried and tested and works well in practice for MPC systems feedback model error to correct the model. This ease of representation of nonlinear plants has made MPC technology widespread, inexpensive to deploy and fast to implement. End-users gain quick benefit realization and return on investments.
Read this case stud on how APC can deal with nonlinearities?